Author: This article is part of an expert series written by Dr. Charbel Rizk, the Founder & CEO of Oculi® - a spinout from Johns Hopkins - a fabless semiconductor startup commercializing patented technology to address the huge inefficiencies with vision technology. In this article, Dr. Rizk discusses the hypothesis that he and his team have developed: Efficient Vision Intelligence (VI) is a prerequisite for effective Artificial Intelligence (AI) for edge applications and beyond.
Despite astronomical advances, human vision remains superior to machine vision and is still our inspiration. The eye is a critical component, which explains the predominance of cameras in AI. With mega-pixels of resolution and trillions of operations per second (TOPS), one would expect vision architecture (camera + computer) today to be on par with human vision. However, current technology is as high as 40,000x behind, particularly in terms of efficiency. It is the combination of the time and energy “wasted” in extracting the required information from the captured signal that is to blame for this inefficiency. This creates a fundamental tradeoff between time and energy, and most solutions optimize one at the expense of the other.

We remain a far cry from replicating the efficacy and speed of human vision. So what is the problem? The answer is surprisingly simple:
-
Cameras and processors operate very differently relative to the human eye and brain, largely because they were historically developed for different purposes. Cameras were built for accurate communication and reproduction. Processors have evolved over time with the primary performance measure being operations per second. The latest trend is domain specific architecture (i.e. custom chips), driven by demand from applications which may see benefit in specialized implementations such as image processing.
-
Another important disconnect, albeit less obvious, is the architecture itself. When a solution is developed from existing components (i.e. off-the-self cameras and processors), it becomes difficult to integrate into a flexible solution and more importantly dynamically optimize in real-time, a key aspect of human vision.
Machine versus Human Vision
To compare, we need to first examine the eyes and brain and the architecture connecting them.
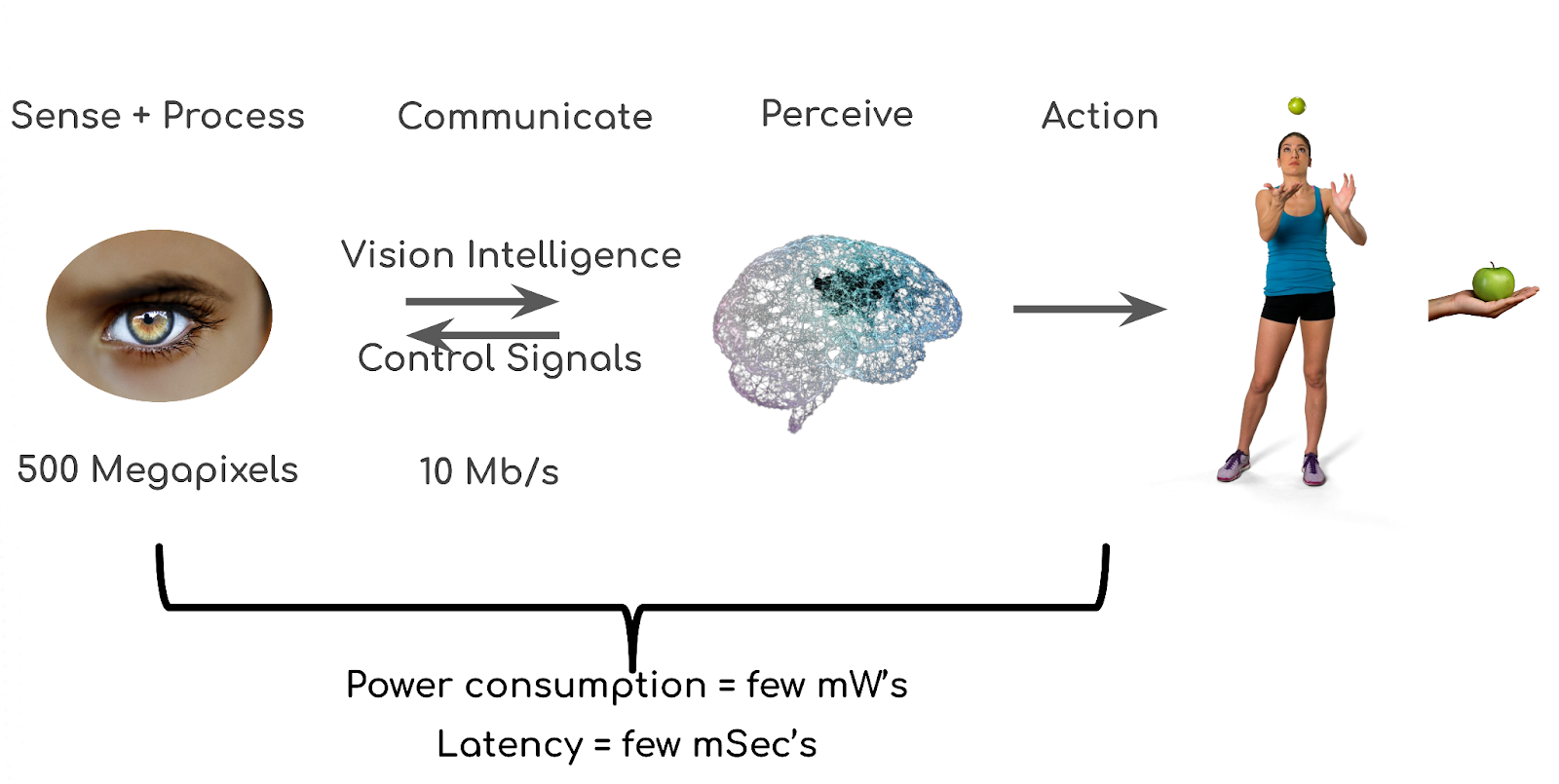
The eye has ~100x more resolution, and if it were operated like a camera it would transfer ~600 Gb/s to the brain. However, the eye-brain “data link” has a maximum capacity of 10 Mbits/sec. So how does it work? The answer is again simple: eyes are specialized sensors which extract and transfer only the “relevant” information (vision intelligence), rather than taking snapshots or videos to store or send to the brain. While cameras are mostly light detectors, the eyes are sophisticated analysts, processing and extracting clues. This sparse but high-yield data is received by the brain for additional processing and eventual perception. Reliable and rapid answers to: What is it?Where is it? and eventually, What does it mean? are the goals of all the processing. The first two questions are largely answered within the eye. The last is answered in the brain. Finally, an important element in efficiency is the communication architecture itself. The eye and the brain are rarely performing the same function at any given moment. There are signals from the brain back to the eye that allow the two organs to continuously optimize and focus on the task at hand.
Efficient Vision Intelligence (VI) is a prerequisite for effective Artificial Intelligence (AI) for edge applications
Everyone is familiar with the term Artificial Intelligence, but what is Vision Intelligence (VI)?
It accurately describes the output of an efficient and truly smart vision sensor like the eye. One that intelligently and efficiently selects and transfers relevant data at a sustainable bandwidth. Biology demonstrates that the eye does a good deal of parallel pre-processing to identify and discard noise (data irrelevant to the task at hand), transferring only essential information. A processing platform that equals the brain is an important step in matching human perception, but not sufficient to achieve human vision without “eye-like” sensors. In the world of vision technology, the human eye represents the power and effectiveness of true edge processing and dynamic sensor optimization.
Efficient Vision Technology is safer and preserves energy
As the world of automation grows exponentially and the demand for imaging sensors skyrockets (cameras being the forerunners with LiDars and radars around the corner), vision technology which is efficient in resources (photon collection, decision time, and power consumption) becomes even more critical to safety and to saving energy.
On safety, a vivid example would be pedestrian detection systems, a critical safety function ripe for an autonomous capability, but current deployed solutions have limited effectiveness. To highlight the challenges with conventional sensors, consider cameras running at 30 frames (or images) per second (fps). That corresponds to a delay of 33 ms to get one image and many are usually required. To get 5 images, the vehicle at 45 mph would have traveled the length of a football field. That “capture” delay can be reduced with increasing the camera speed (more images per second) but that creates other challenges in sensor sensitivity and/or system complexity. In addition, night time operation presents its own unique challenges and those challenges increase with the sampling speed.
Real-time processing would also be necessary to not add more delay to the system. Two HD cameras generate about 2 Gbits/sec. This data rate, when combined with the associated memory and processing, causes the overall power consumption for real-time applications to become significant. Some may assume that a vehicle has an unlimited energy supply. But often that is not the case. In fact, some fossil fuel vehicle companies are having to upsize their vehicles’ engines due to the increased electric power consumption associated with ADAS. Moreover, with the world moving towards electric vehicles, every watt counts.
If we were to think beyond our edge applications and look at the power cost of inefficient vision technology in general, the findings may surprise the reader. Recent studies estimate that a single email costs 4 grams of CO2 emission and 50g if it includes a picture, which is exactly the problem with vision technology today. It produces too much data. If we consider a typical vision system (camera+network+storage+processing) and assume, conservatively, a total power consumption of 5 Watts and that roughly 1 billion cameras are on at any given time, this translates to a total power consumption of 44 Terawatt-hr/yr. This is more than 163 out of 218 countries and territories, or mid-way between the power consumption of Massachusetts and Nevada. In the age of data centers, images, and videos, “electronics” will soon become the dominant energy consumers and sources of carbon emissions in the future.
Machine vision is never about capturing pretty pictures but it needs to generate the “best” actionable information very efficiently from the available signal (photons). What this means is optimizing the architecture on edge applications, which by nature, are resource constrained. This is exactly what nature provided in human vision. Biological organs such as the human eyes and brain operate at performance levels set by fundamental physical limits, under severe constraints of size, weight, and energy resources—the same constraints that tomorrow’s edge solutions have to meet.
There is significant room for improvement still by simply optimizing the architecture of current machine vision applications, in particular the signal processing chain from capture to action, and human vision is a perfect example of what’s possible. Before the world jumps to adding additional sensors to the mix, the focus should be on structuring the system in an optimal way to allow for the power of machine vision to approach that of human vision.